W
ith the growing number of tigers inhabiting Bardiya National Park (BNP) an increasingly relevant question is how much ‘food’ these tigers need. In other words: does BNP have a sufficient prey base to sustain the tiger population currently, and in the future?
The PhD research of Shyam Thapa focusses on generating a better understanding of grasslands within the context of BNP. These grasslands are maintained by grazing herbivores that in turn also serve as important food supply. The missing link between grass and tiger is the population of species that are dependent on grasslands and prey for tiger, consisting of species such as spotted deer (Axis axis), sambar deer (Rusa unicolor) and wild boar (Sus scrofa).
Currently, local observations and the 2018 census by NTNC seem to indicate that the population of prey species is declining in BNP (See: https://ntnc.org.np/publication/status-tigers-and-prey-nepal-2018). This raises several questions:
1. How many ungulates are needed to maintain grassland in the state of grazing lawn, with highly nutritious grasses?
2. What population of prey species is needed to sustain the current, and growing, tiger population in BNP?
3. What is a sustainable tiger population in BNP, with respect to the prey base?
4. Is BNP at risk of a predator pit,where a high predator-prey ratio leads to the predators eating more prey than are added by reproduction, eventually leading to a depletion in prey and subsequent crash in predators.
In short: we need a better understanding of the population of prey animals in BNP. This is traditionally done by visually counting animals. Over time, new methods such as line transects were developed, but traditional methods that rely on a human observer have several major drawbacks. First, it is labor intensive and requires many hours in the field for just a single estimate. Dynamics in space and time are thus difficult to obtain. Second, rare or illusive species tend to be missed or underestimated. Third, differences between persons introduce a bias. One person may be much more adept at spotting animals than the other.
In recent years motion-sensitive camera traps have become an accepted method of estimating animal populations. As a non-invasive method, they greatly reduce the biases inherent to human observers and field labor needed to obtain data. Because camera traps can function independently for weeks at a time, continuous monitoring of population comes within reach. From a statistical point of view working with camera traps has some challenges. Traditionally, camera traps are aimed parallel to the ground, which although practical, means that complicated mathematics are needed to translate photos into meaningful numbers. In this study, we will pioneer a completely novel setup: cameras are placed at a height of 8 meters and aimed vertically downwards. This greatly simplifies the statistics and will lead to more accurate estimates.
See: preparation installation of a camera trap at eight meters height (video YouTube)
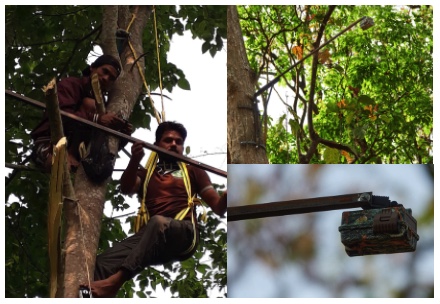
Umesh Paudel and colleague install a camera arm. The novel method of vertical placement of camera traps is pioneered in BNP.
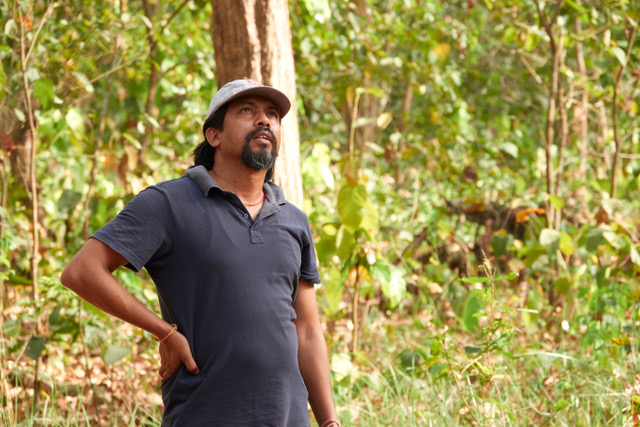
Shyam Thapa supervises the installation of the cameras.
NTNC, in close collaboration with the Department of National Parks Wildlife Conservation (DNSWC) and Wageningen University (WUR) and financially supported by HTF, is establishing a permanent network of 50 camera traps in BNP. With this network, the entire community of terrestrial mammals will be monitored continuously. The network will operate for the coming years, yielding more accurate data on the population dynamics of prey species and tiger.
Photos collected by the cameras will be analyzed with the help of artificial intelligence, which can reliably extract the species and number of animals present on each photo. The first insights in the dynamics of tiger and prey in BNP are expected by the end of 2019.
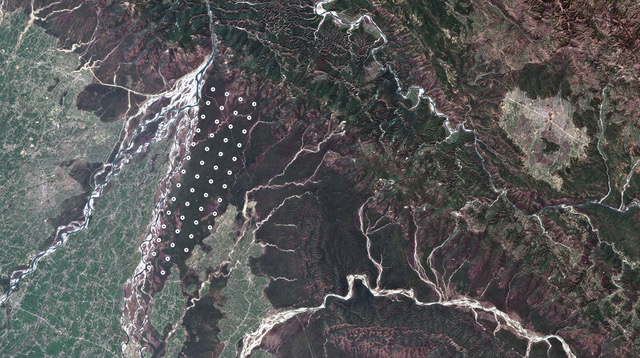
The network of camera traps will cover Western BNP, and operate continuously.
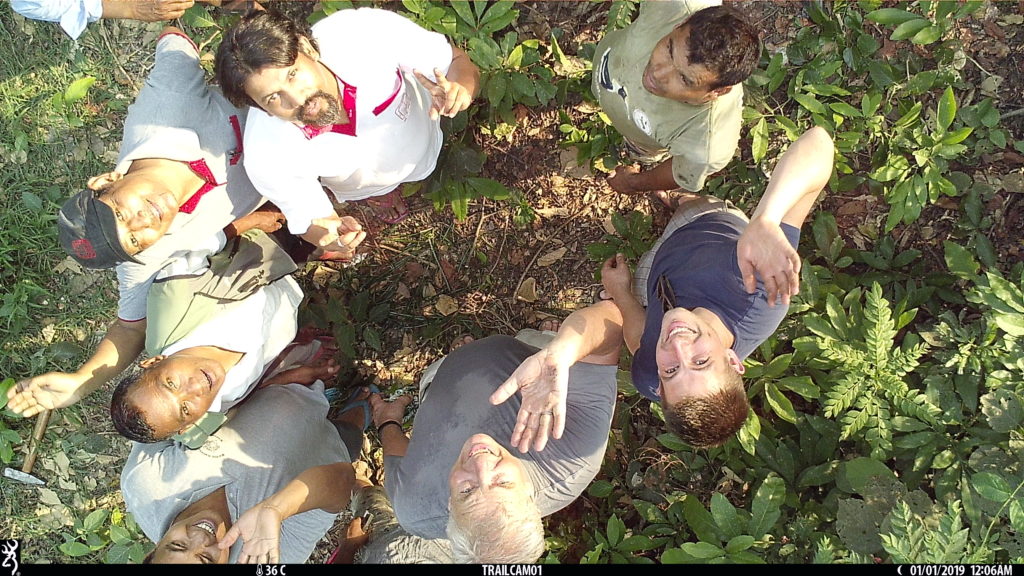
The team consisting of members of NTNC, HTF and WUR after installing the first camera trap.